class: center middle main-title section-title-6 # DAGs and the<br>Causal Revolution --- layout: true class: title title-6 --- # Types of data .pull-left[ .box-inv-6.medium[Experimental] .box-6[You have control over which units get treatment] ] -- .pull-right[ .box-inv-6.medium[Observational] .box-6[You don't have control over which units get treatment] ] -- .box-inv-6.medium.sp-before[Which kind lets you prove causation?] --- # Causation with observational data .box-inv-6.medium.sp-after[Can you prove causation<br>with observational data?] -- .box-inv-6.medium[Why is it so controversial<br>to use observational data?] --- # The causal revolution .pull-left-narrow[ <figure> <img src="img/01/book-of-why.jpg" alt="The Book of Why" title="The Book of Why" width="100%"> </figure> ] .pull-right-wide.center[ <figure> <img src="img/01/judea-pearl.jpg" alt="Judea Pearl" title="Judea Pearl" width="90%"> </figure> ] --- # Causal diagrams .box-inv-6.medium[Directed acyclic graphs (DAGs)] .pull-left[ .box-6.SMALL[Graphical model of the process that generates the data] .box-6.SMALL[Maps your philosophical model] .box-6.SMALL[Fancy math ("*do*-calculus") tells you what to control for to isolate and identify causation] ] .pull-right[ <img src="01_dags_files/figure-html/simple-dag-1.png" width="100%" style="display: block; margin: auto;" /> ] --- # How to draw a DAG .box-inv-6.medium[What is the causal effect of an<br>additional year of education on earnings?] -- .box-6[Step 1: List variables] -- .box-6[Step 2: Simplify] -- .box-6[Step 3: Connect arrows] -- .box-6[Step 4: Use logic and math to determine<br>which nodes and arrows to measure] --- # 1. List variables .box-6.sp-after[Education (treatment) → Earnings (outcome)] .center.float-left.sp-after-half[ .box-inv-6[Location] .box-inv-6[Ability] .box-inv-6[Demographics] ] .center.float-left.sp-after-half[ .box-inv-6[Socioeonomic status] .box-inv-6[Year of birth] ] .center.float-left[ .box-inv-6[Compulsory schooling laws] .box-inv-6[Job connections] ] --- # 2. Simplify .box-6.sp-after[Education (treatment) → Earnings (outcome)] .center.float-left.sp-after-half[ .box-inv-6[Location] .box-inv-3[Ability] .box-inv-3[Demographics] ] .center.float-left.sp-after-half[ .box-inv-3[Socioeonomic status] .box-inv-6[Year of birth] ] .center.float-left[ .box-inv-6[Compulsory schooling laws] .box-inv-6[Job connections] ] .box-inv-5[Background] --- # 3. Draw arrows .pull-left-narrow[ .box-inv-6[Education causes earnings] ] .pull-right-wide[ <img src="01_dags_files/figure-html/edu-earn-simple-1.png" width="100%" style="display: block; margin: auto;" /> ] --- # 3. Draw arrows .pull-left-narrow[ .box-inv-6[Background, year of birth, location, job connections, and school requirements all cause education] ] .pull-right-wide[ <img src="01_dags_files/figure-html/edu-earn-2-1.png" width="100%" style="display: block; margin: auto;" /> ] --- # 3. Draw arrows .pull-left-narrow[ .box-inv-6[Background, year of birth, and location all cause earnings too] ] .pull-right-wide[ <img src="01_dags_files/figure-html/edu-earn-3-1.png" width="100%" style="display: block; margin: auto;" /> ] --- # 3. Draw arrows .pull-left-narrow[ .box-inv-6[Education causes job earnings] ] .pull-right-wide[ <img src="01_dags_files/figure-html/edu-earn-4-1.png" width="100%" style="display: block; margin: auto;" /> ] --- # 3. Draw arrows .pull-left-narrow[ .box-inv-6[Location and background are probably related, but neither causes the other. Something unobservable (U1) does that.] ] .pull-right-wide[ <img src="01_dags_files/figure-html/edu-earn-full-1.png" width="100%" style="display: block; margin: auto;" /> ] --- layout: false class: title title-6 section-title-inv-6 # Your turn #1 .box-inv-6.medium[Does a longer night's sleep<br>extend your lifespan?] .box-6[Step 1: List variables] .box-6[Step 2: Simplify] .box-6[Step 3: Connect arrows] .box-6[Use dagitty.net]
05
:
00
--- layout: true class: title title-6 --- # Causal identification .pull-left-narrow[ .box-inv-6[All these nodes are related; there's correlation between them all] .box-inv-6[We care about Edu → Earn, but what do we do about all the other nodes?] ] .pull-right-wide[ 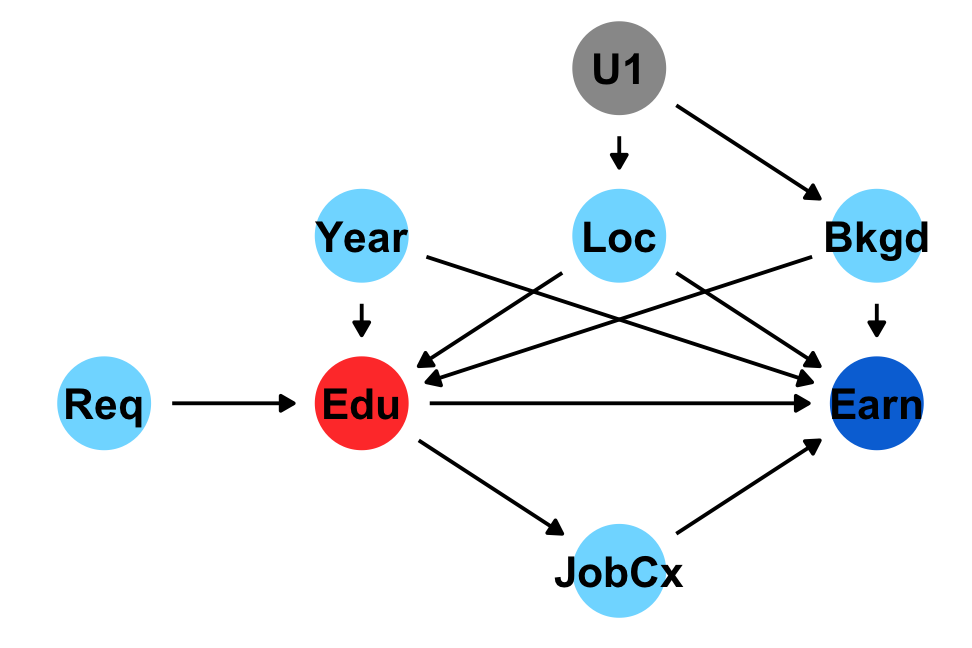 ] --- # Causal identification .box-inv-6.medium[A causal effect is *identified* if the association between treatment and outcome is propertly stripped and isolated] --- # Paths and associations .box-inv-6.medium[Arrows in a DAG transmit associations] .box-inv-6.medium[You can redirect and control those paths by "adjusting" or "conditioning"] --- # Three types of associations .pull-left-3[ .box-6.medium[Confounding] <img src="01_dags_files/figure-html/confounding-dag-1.png" width="100%" style="display: block; margin: auto;" /> .box-inv-6.small[Common cause] ] .pull-middle-3[ .box-6.medium[Causation] <img src="01_dags_files/figure-html/mediation-dag-1.png" width="100%" style="display: block; margin: auto;" /> .box-inv-6.small[Mediation] ] .pull-right-3[ .box-6.medium[Collision] <img src="01_dags_files/figure-html/collision-dag-1.png" width="100%" style="display: block; margin: auto;" /> .box-inv-6.small[Selection /<br>endogeneity] ] --- # Confounding .pull-left-wide[ <img src="01_dags_files/figure-html/confounding-dag-big-1.png" width="100%" style="display: block; margin: auto;" /> ] .pull-right-narrow[ .box-inv-6.medium[**X** causes **Y**] .box-inv-6.medium[But **Z** causes both **X** and **Y**] .box-inv-6.medium[**Z** * confounds* the **X** → **Y** association] ] --- # Paths .pull-left-wide[ 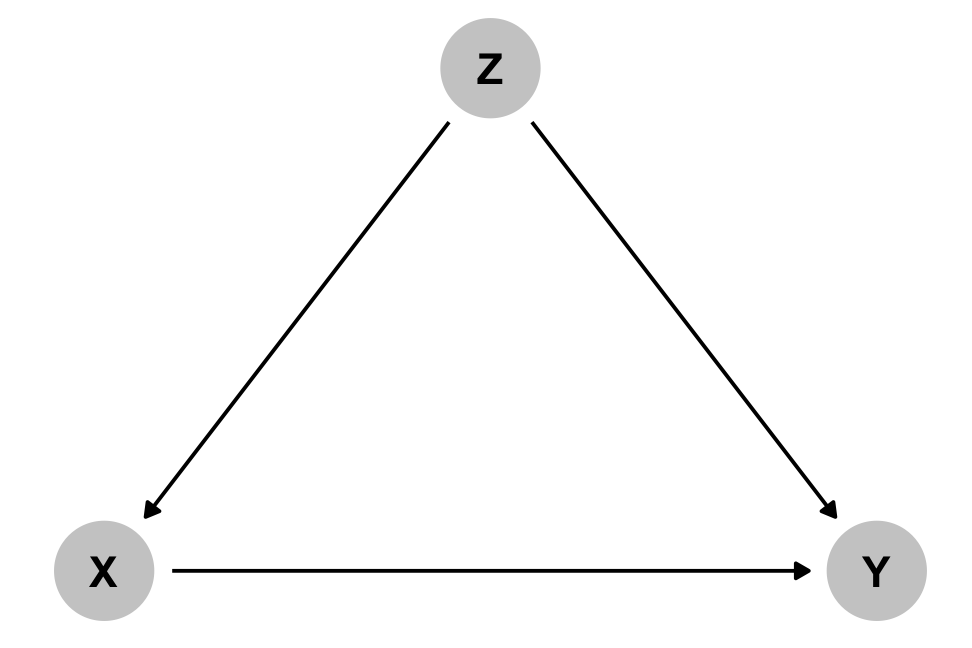 ] .pull-right-narrow[ .box-inv-6[Paths between<br>**X** and **Y**?] .box-6[**X** → **Y**] .box-6[**X** ← **Z** → **Y**] .box-inv-6.medium[**Z** is a *backdoor*] ] --- # *d*-connection .pull-left-wide[ 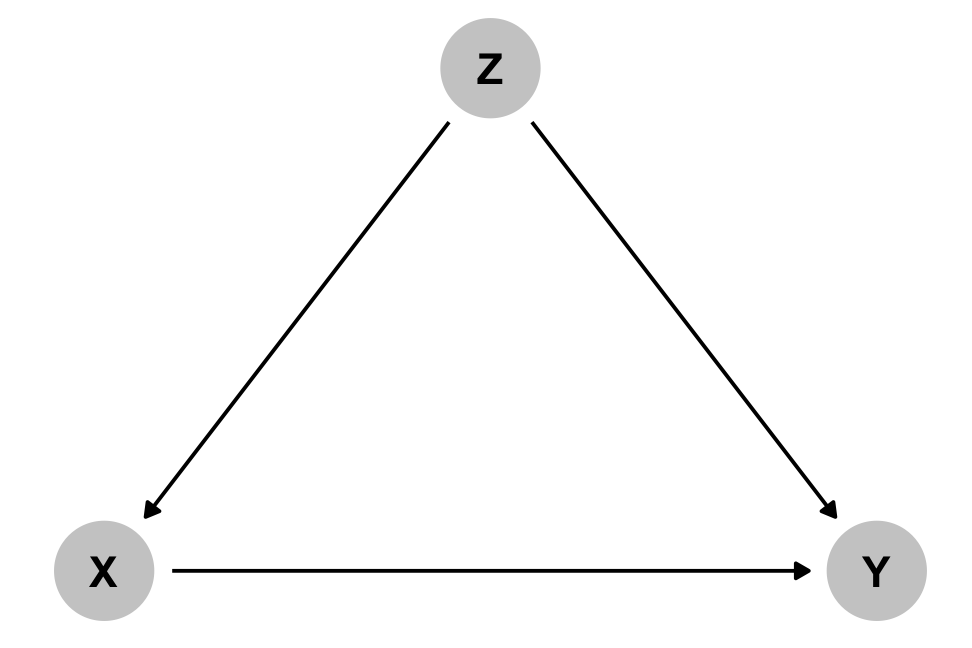 ] .pull-right-narrow[ .box-inv-6[**X** and **Y** are "*d*-connected" because associations can pass through **Z**] .box-6[The relationship between **X** and **Y** is not identified / isolated] ] --- # Effect of money on elections .box-inv-6.medium[What are the paths<br>between **money** and **win margin**?] .pull-left[ <img src="01_dags_files/figure-html/money-elections-1.png" width="100%" style="display: block; margin: auto;" /> ] -- .pull-right[ .box-6[Money → Margin] .box-6[Money ← Quality → Margin] .box-inv-6[Quality is a *backdoor*] ] --- # Closing doors .pull-left[ <img src="01_dags_files/figure-html/confounding-dag-adjusted-1.png" width="100%" style="display: block; margin: auto;" /> ] .pull-right[ .box-inv-6.medium[Close the backdoor by adjusting for **Z**] ] --- # Closing doors .pull-left[ .box-inv-6.small[Find the part of campaign money that is explained by quality, subtract it out.<br>This is the residual part of money.] .box-inv-6.small[Find the part of win margin that is explained by quality, subtract it out. This is the residual part of win margin.] .box-inv-6.small[Find the relationship between the residual part of money and residual part of win margin.<br>**This is the causal effect**.] ] .pull-right[ <img src="01_dags_files/figure-html/money-elections-adjusted-1.png" width="100%" style="display: block; margin: auto;" /> ] --- # Closing doors .pull-left[ .box-inv-6[Compare candidates as if they had the same quality] .box-inv-6[Remove differences that are predicted by quality] .box-inv-6[Hold quality constant] ] .pull-right[ 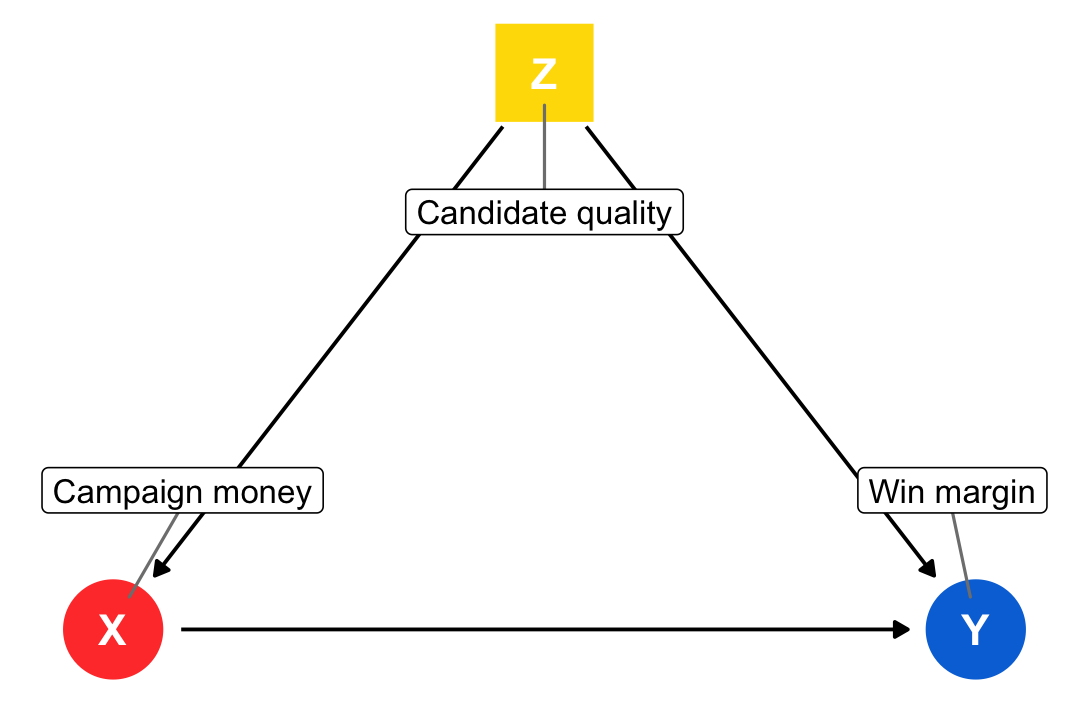 ] --- # How to adjust .box-inv-6.medium[Include term in regression] $$ `\begin{aligned} \text{Win margin} =& \beta_0 + \beta_1 \text{Campaign money} +\\ & \beta_2 \text{Candidate quality} + \varepsilon \end{aligned}` $$ .center.float-left[ .box-inv-6.medium[Matching] .box-inv-6.medium[Stratifying] .box-inv-6.medium.sp-before-half[Inverse probability weighting] ] --- # *d*-separation .pull-left[ 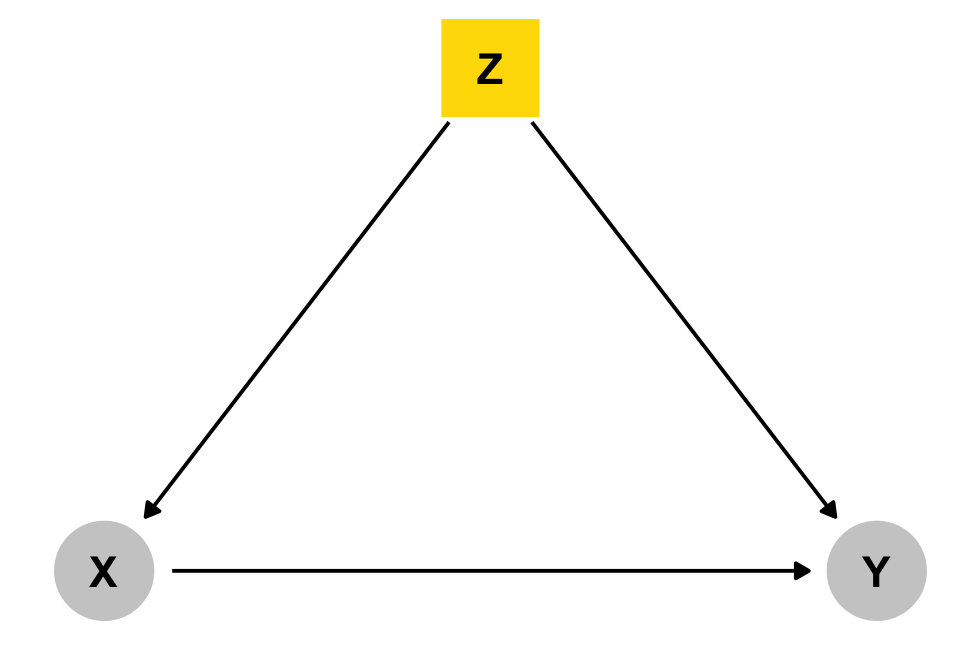 ] .pull-right[ .box-inv-6.medium[If we control for **Z**,<br>**X** and **Y** are now<br>"*d*-separated" and the association is isolated!] ] --- # Closing backdoors .pull-left-narrow[ .box-inv-6[Block all backdoor paths to identify the main pathway you care about] ] .pull-right-wide[ 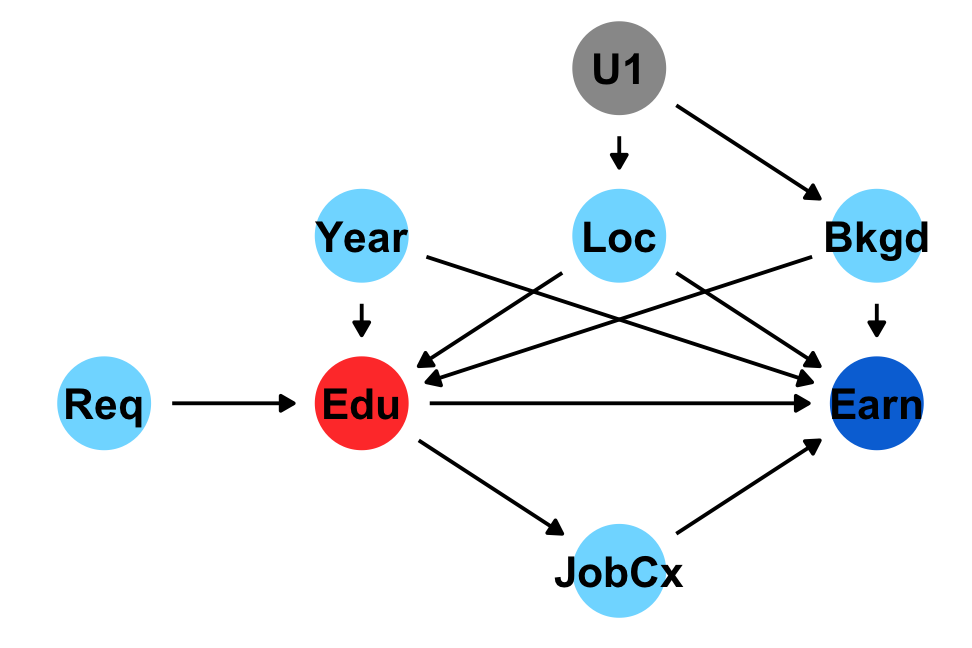 ] --- # All paths .pull-left.left[ .box-6.smaller[Education → Earnings] .box-6.smaller[Education → Job connections → Earnings] .box-2.smaller[Education ← Background → Earnings] .box-2.smaller[Education ← Background ← U1 → Location → Earnings] .box-2.smaller[Education ← Location → Earnings] .box-2.smaller[Education ← Location ← U1 → Background → Earnings] .box-2.smaller[Education ← Year → Earnings] ] .pull-right[ 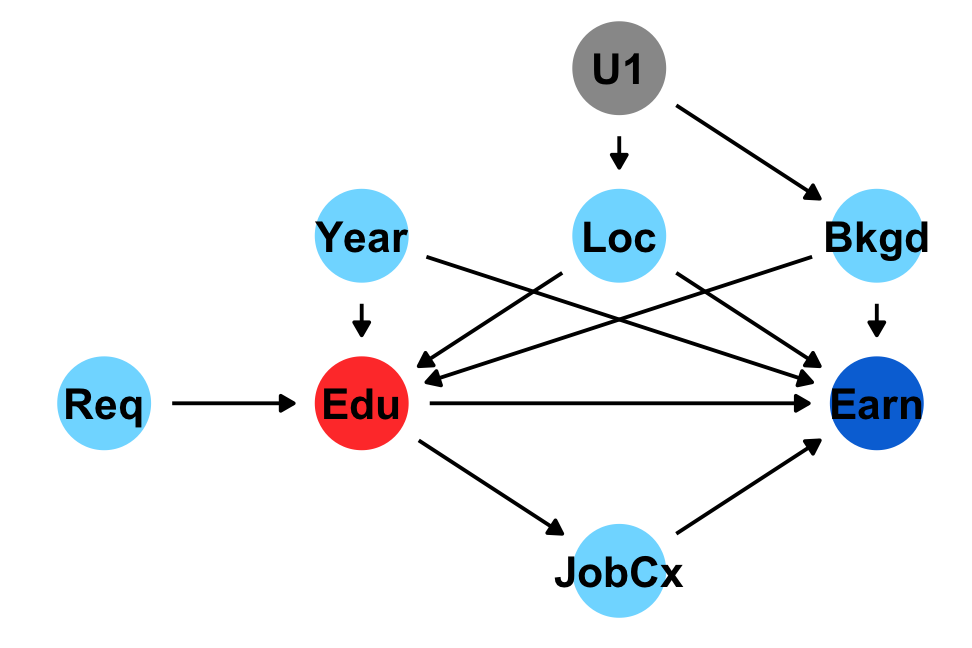 ] --- # All paths .pull-left-narrow[ .box-inv-6[Adjust for **Location**, **Background** and **Year** to isolate the **Education → Earnings** causal effect] ] .pull-right-wide[ <img src="01_dags_files/figure-html/edu-earn-adjust-1.png" width="100%" style="display: block; margin: auto;" /> ] --- # Let the computer do this! .box-inv-6.large[dagitty.net] --- # How do you know if this is right? .pull-left-narrow[ .box-inv-6[You can test the implications of the model to see if they're right in your data] $$ X \perp Y\ |\ Z $$ .box-6.small[X is independent of Y, given Z] ] .pull-right-wide[ <figure> <img src="img/01/dagitty-implications.png" alt="Dagitty implications" title="Dagitty implications" width="100%"> </figure> ] --- layout: false class: title title-6 section-title-inv-6 # Your turn #2 .box-6.medium[Go to andhs.co/nyt and skim the article] .box-6.medium[Pick one of the causal claims in the article] .box-6.medium[Draw a DAG for that causal claim] .box-6.medium[Determine what needs to be<br>adjusted to identify the effect]
06
:
00
--- layout: true class: title title-6 --- # Causation .pull-left-wide[ <img src="01_dags_files/figure-html/causation-dag-big-1.png" width="100%" style="display: block; margin: auto;" /> ] .pull-right-narrow[ .box-inv-6.medium[**X** causes **Y**] .box-inv-6.medium[**X** causes<br>**Z** which causes **Y**] .box-6.medium[Should you control for **Z**?] ] --- # Causation .pull-left-wide[ 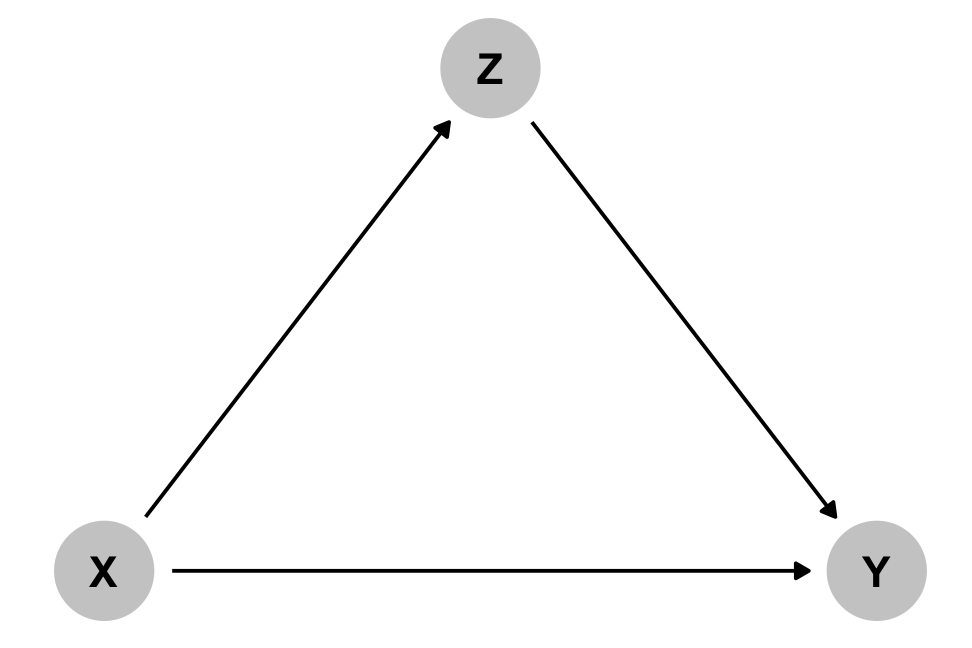 ] .pull-right-narrow[ .box-6.medium[Should you control for **Z**?] .box-inv-6.medium[No!] .box-inv-6[Overcontrolling] ] --- # Causation and overcontrolling .pull-left-wide[ 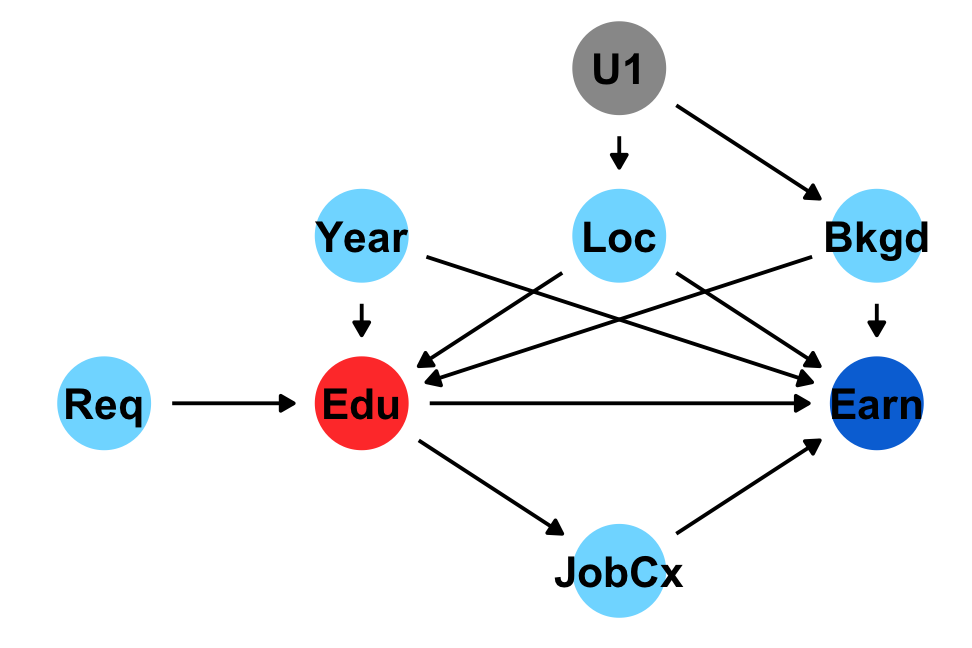 ] .pull-right-narrow[ .box-inv-6[Should you control for job connections?] ] --- # Colliders .pull-left-wide[ <img src="01_dags_files/figure-html/collider-dag-big-1.png" width="100%" style="display: block; margin: auto;" /> ] .pull-right-narrow[ .box-inv-6.medium[**X** causes **Z**] .box-inv-6.medium[**Y** causes **Z**] .box-6.medium[Should you control for **Z**?] ] --- # Programming and social skills .box-inv-6.medium[Do programming skills reduce social skills?] .pull-left[ <img src="01_dags_files/figure-html/programming-social-skills-1.png" width="100%" style="display: block; margin: auto;" /> ] -- .pull-right[ .box-6[You go to a tech company and conduct a survey. You find a negative relationship!<br>Is it real?] ] --- # Programming and social skills .box-inv-6.medium[Do programming skills reduce social skills?] .pull-left[ 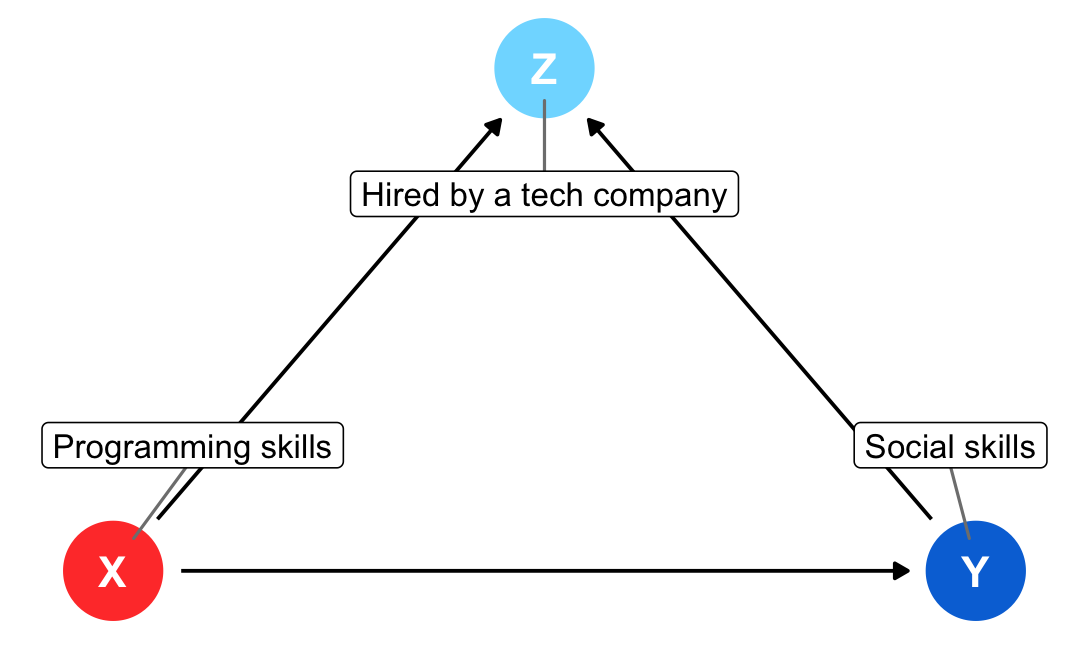 ] .pull-right[ .box-6[No! **Hired by a tech company** is a collider and we controlled for it.] .box-6[This inadvertently connected the two.] ] --- layout: false .pull-left[ .box-6.medium[Colliders can create<br>fake causal effects] ] .pull-right[ .box-6.medium[Colliders can hide<br>real causal effects] ] <img src="01_dags_files/figure-html/bulls-scores-1.png" width="50%" style="display: block; margin: auto;" /> .center[ .box-inv-6[Height is unrelated to basketball skill… among NBA players] ] --- layout: true class: title title-6 --- # Colliders and selection bias <img src="01_dags_files/figure-html/nba-dag-1.png" width="65%" style="display: block; margin: auto;" /> --- # Three types of associations .pull-left-3[ .box-6.medium[Confounding] 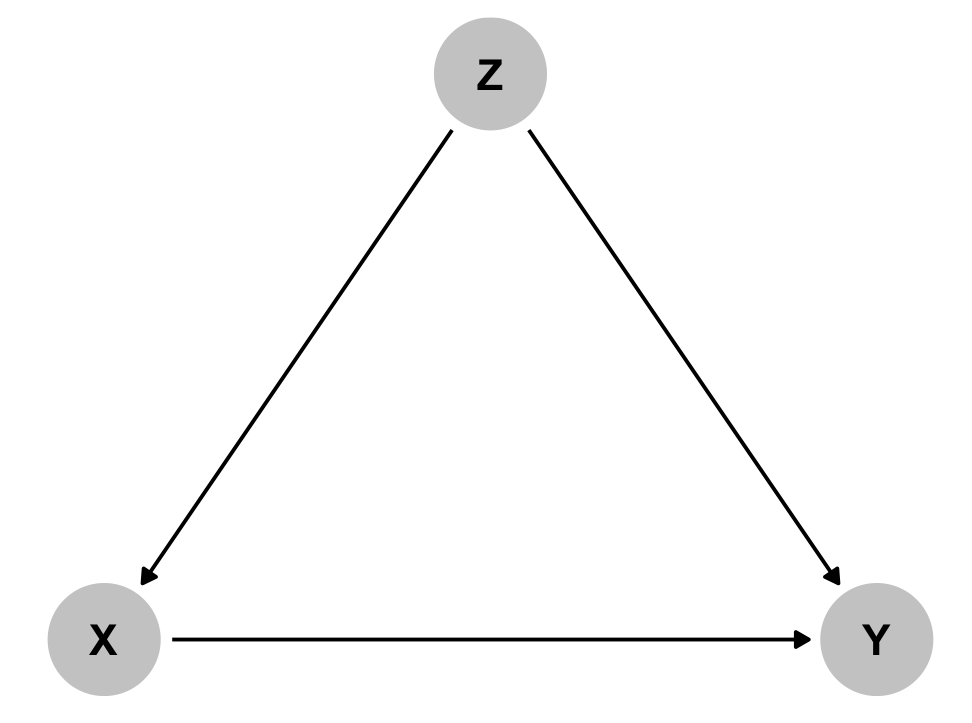 .box-inv-6.small[Common cause] ] .pull-middle-3[ .box-6.medium[Causation] 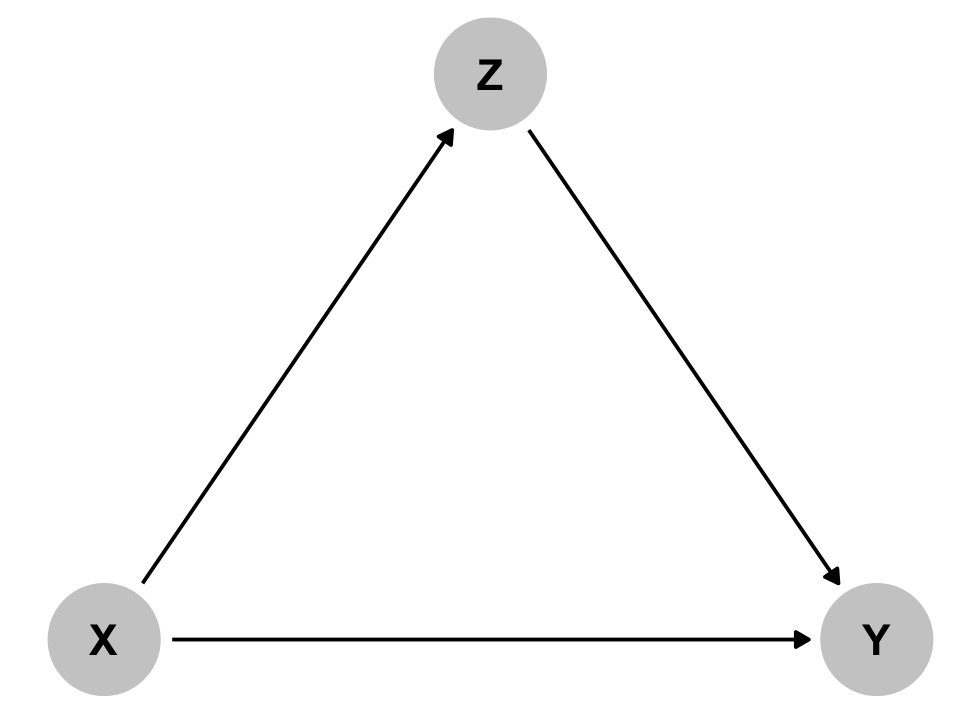 .box-inv-6.small[Mediation] ] .pull-right-3[ .box-6.medium[Collision] 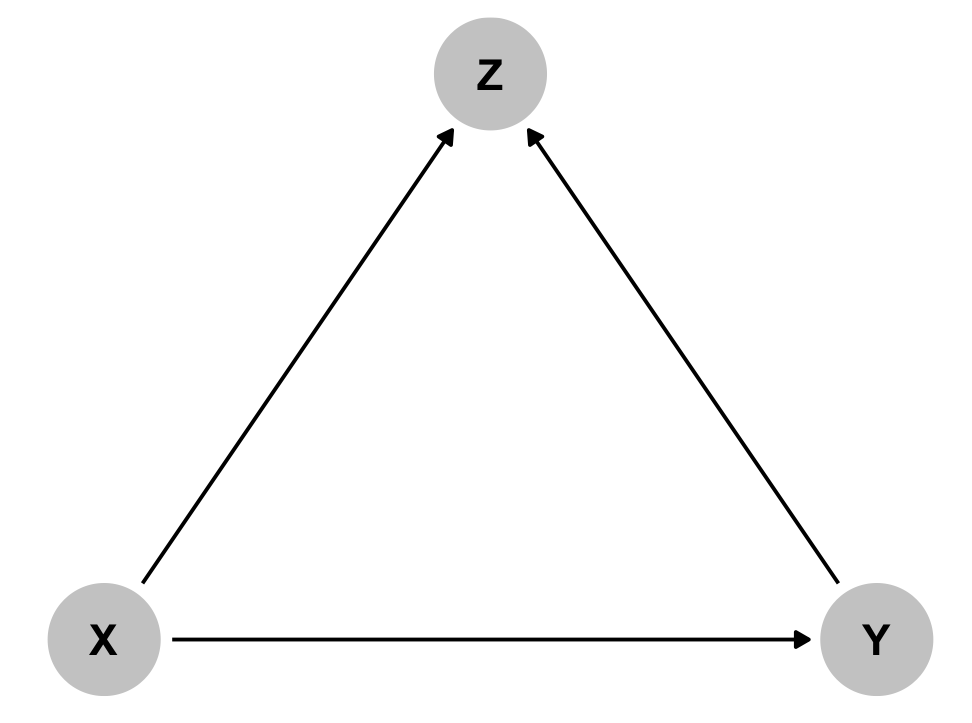 .box-inv-6.small[Selection /<br>endogeneity] ] --- # Next up .box-inv-6.medium[How to analyze RCTs]